- Low-carbon cities
- Smart cities
- pilots for pleasant and healthy green environment
Artificial intelligence and machine vision methods in improving regional land cover classification
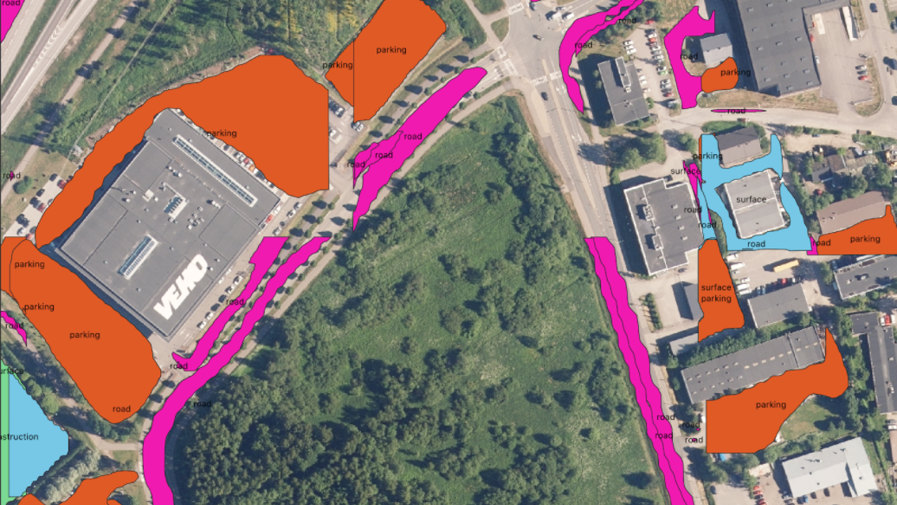
Cities need information on the location of water-permeable and impermeable surfaces in their territory for land planning, flood risk analyses and simulations.
We aimed to develop AI and machine vision based land cover classification methods for HSY’s regional remote sensing data.
In particular, we focused on automatic identification and mapping of surfaces (asphalt, sand) that are essential for storm water management/permeability from aerial images.
Results
- The expectations were not met, as the tested starting material was not sufficient for automatic classification.
- New experiences were gained in the use of the Mask-RCNN neural network and Tensorflow for a new theme.
- An understanding of the significance of adequate starting material and precise classification and the amount of teaching material.
- An understanding of the challenges related to the AI/NN process and setting performance expectations at a realistic level.
Effects
- The experiment did not result in any direct effects.
- If it had been successful, the method could have been widely utilised in Finnish municipalities and internationally.
- Specifying and adding source data would have a significant impact on the success of automatic classifications. The method in itself does work, but requires better basic data.
In collaboration with
- Pointscene Oy
- Helsinki Region Environmental Services HSY
- Ministry of the Environment